| C2AM Loss: Chasing a Better Decision Boundary for Long-Tail Object Detection |
| Wang, Tong1,4; Zhu, Yousong1; Chen, Yingying1; Zhao, Chaoyang1,3; Yu, Bin1,4; Wang, Jinqiao1,2,4; Tang, Ming1
|
| 2022-06
|
会议日期 | 2022-6-19
|
会议地点 | New Orleans, Louisiana & Online
|
英文摘要 | Long-tail object detection suffers from poor performance
on tail categories. We reveal that the real culprit lies in the
extremely imbalanced distribution of the classifier’s weight
norm. For conventional softmax cross-entropy loss, such
imbalanced weight norm distribution yields ill conditioned
decision boundary for categories which have small weight
norms. To get rid of this situation, we choose to maximize the cosine similarity between the learned feature and
the weight vector of target category rather than the innerproduct of them. The decision boundary between any two
categories is the angular bisector of their weight vectors.
Whereas, the absolutely equal decision boundary is sub
optimal because it reduces the model’s sensitivity to vari
ous categories. Intuitively, categories with rich data diver
sity should occupy a larger area in the classification space
while categories with limited data diversity should occupy
a slightly small space. Hence, we devise a Category-Aware
Angular Margin Loss (C2AM Loss) to introduce an adap
tive angular margin between any two categories. Specif
ically, the margin between two categories is proportional
to the ratio of their classifiers’ weight norms. As a result,
the decision boundary is slightly pushed towards the cat
egory which has a smaller weight norm. We conduct com
prehensive experiments on LVIS dataset. C2AM Loss brings
4.9∼5.2 AP improvements on different detectors and back
bones compared with baseline. |
|
内容类型 | 会议论文
|
源URL | [http://ir.ia.ac.cn/handle/173211/47418] 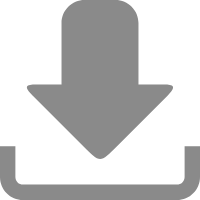 |
专题 | 自动化研究所_模式识别国家重点实验室_图像与视频分析团队
|
作者单位 | 1.National Laboratory of Pattern Recognition, Institute of Automation, Chinese Academy of Sciences, Beijing, China 2.Peng Cheng Laboratory, Shenzhen, China 3.Development Research Institute of Guangzhou Smart City, Guangzhou, China 4.School of Artificial Intelligence, University of Chinese Academy of Sciences, Beijing, China
|
推荐引用方式 GB/T 7714 |
Wang, Tong,Zhu, Yousong,Chen, Yingying,et al. C2AM Loss: Chasing a Better Decision Boundary for Long-Tail Object Detection[C]. 见:. New Orleans, Louisiana & Online. 2022-6-19.
|
修改评论